Adaptive Models Capture Complexity of the Brain and Behavior
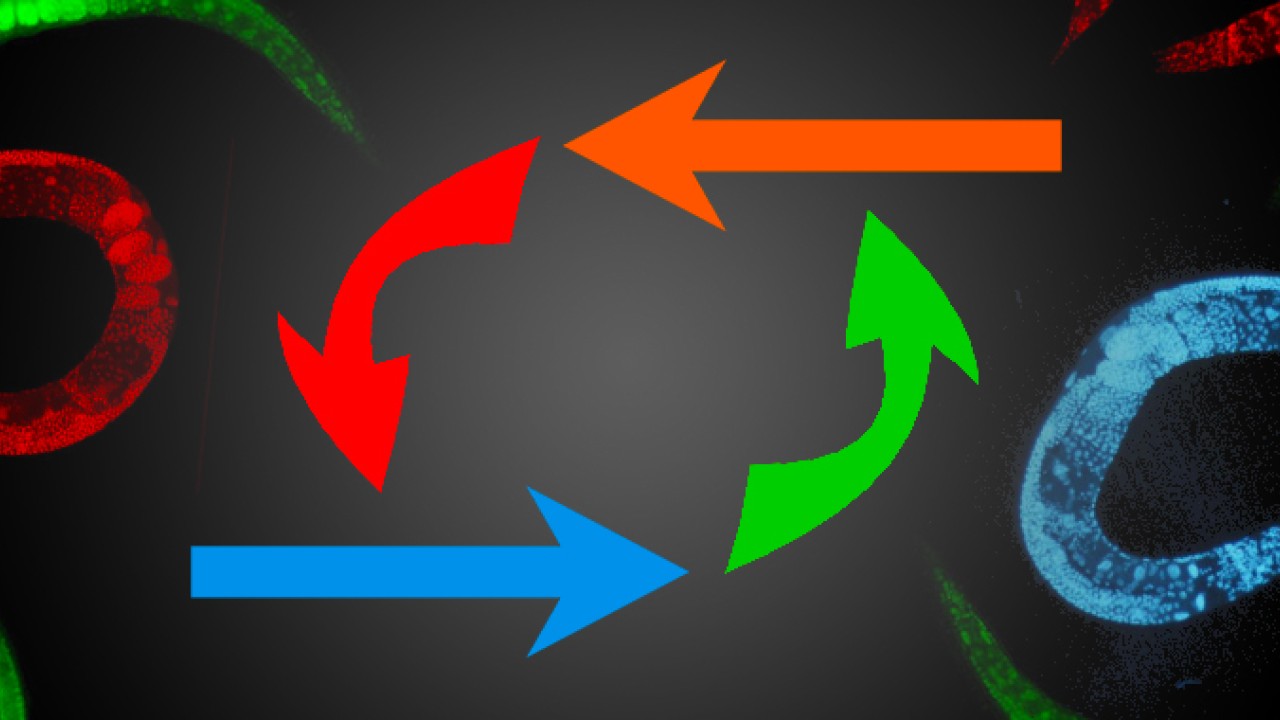
For the scientists that study animal behavior, even the simplest roundworm poses huge challenges. The movement of squirming worms, flocking birds and walking humans changes from moment to moment, in ways that the naked eye can’t catch. But now, researchers from the Okinawa Institute of Science and Technology Graduate University (OIST) and Vrije Universiteit Amsterdam have developed a way to parse this dynamic behavior into digestible chunks.
“Even if you just want to classify movement as moving forward, backward, or turning, you can’t be sure just by eye,” said Tosif Ahamed, an author of the study and graduate student in the OIST Biological Physics Theory Unit, led by Prof. Greg Stephens, as well as the Information Processing Biology Unit led by Prof. Ichiro Maruyama. By handing the observation over to an adaptive model, the researchers spotted subtleties they would have otherwise missed. “With this method, we don’t have to throw away any details.”
The study, published online January 17, 2019 in the Proceedings of the National Academy of Sciences of the United States of America, found that complex dynamics can be broken down into a collection of simple linear patterns. The researchers diced their data into distinct time windows based on how these patterns changed over time. By clustering time windows that appeared statistically similar, the model revealed distinct patterns in animals’ changing brain states and movement behaviors.
“You make only minimal assumptions from the start,” said Antonio C. Costa, first author of the paper and graduate student in the Department of Physics and Astronomy at Vrije Universiteit Amsterdam. “You can let the data tell you what the animal is doing. This can be powerful...and allow you to find new classes of behavior.”
Crawling - Not as Simple as it Looks
The model uncovered rich complexity underlying one of the simplest of movements: namely, crawling. Scientists can observe Caenorhabditis elegans as the worm wriggles forward, turns, or reverses its motion to crawl backward. These behaviors appear simple, but upon closer inspection, each movement contains its own variety and nuance.
There’s more than one way to crawl.
“We knew implicitly, by watching the worms, about these coarse behavioral categories.
But they’re not that simple,” said Prof. Stephens, who also holds a position at Vrije Universiteit Amsterdam. “There are more subtle behavioral states you might not see by eye.”
The data suggest that C. elegans remains poised and ready to switch behaviors at a moment’s notice. Like agile boxers, primed to bob or weave in response to their opponent’s next jab, the worms’ movement hovers on the edge of one pattern and the next. Prior research suggests that more complex creatures, such as humans, also display this adaptability. The new modeling technique allows scientists to quantify these dynamics directly.
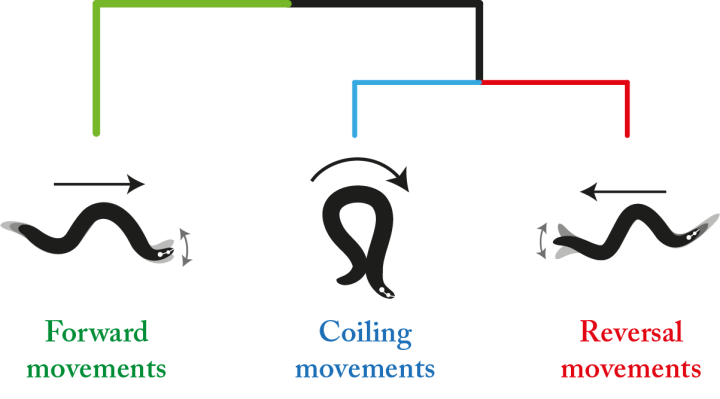
Applications Beyond Behavior
Besides modeling behavior in C. elegans, the researchers also quantified whole brain dynamics in the worm, in neurons from the visual cortex of mice, and in the cerebral cortex of monkeys.
“It was surprising—ours is a simple approach, but it proved powerful for interpreting this variety of complex systems,” said Stephens. Dynamical systems crop up everywhere in nature, not just in the brain. Fluid mechanics, turbulence and even the collective movement of flocking birds exemplify systems that could be decoded using the new approach. This idea could also be combined with machine learning methods to classify videos as we do still images, which remains a major challenge in the field.
“Once you can describe dynamics in a principled way, you can apply the technique to many systems.”
Specialty
Research Unit
For press enquiries:
Press Inquiry Form