研究ユニット
教員・研究ユニットを探す
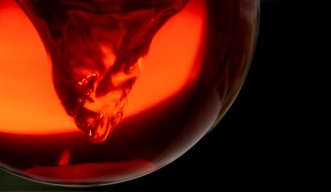
生体制御分子創製化学ユニット
生体制御分子創製化学ユニットでは、生命機能の解明や創薬に貢献する分子の創製に関する研究を行なっています。研究領域は、有機合成化学、生物有機化学です。

田中 富士枝
教授
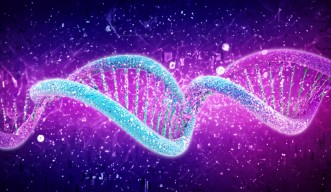
生態・進化ゲノミクス アルゴリズムユニット
生態・進化ゲノミクスアルゴリズムユニットでは、地球上の現存する種のゲノムの組立てや比較などを研究し、ゲノミクスのコア問題の為のアルゴリズムを開発します。

ジーン・マイヤーズ
教授(アジャンクト)

生物の非線形力学データサイエンス研究ユニット
The biological nonlinear dynamics data science unit investigates complex systems explicitly taking into account the role of time. We do this by instead of averaging occurrences using their statistics, we treat observations as frames of a movie and if patterns reoccur then we can use their behaviors in the past to predict their future. In most cases the systems that we study are part of complex networks of interactions and cover multiple scales. These include but are not limited to systems neuroscience, gene expression, posttranscriptional regulatory processes, to ecology, but also include societal and economic systems that have complex interdependencies. The processes that we are most interested in are those where the data has a particular geometry known as low dimensional manifolds. These are geometrical objects generated from embeddings of data that allows us to predict their future behaviors, investigate causal relationships, find if a system is becoming unstable, find early warning signs of critical transitions or catastrophes and more. Our computational approaches are based on tools that have their origin in the generalized Takens theorem, and are collectively known as empirical dynamic modeling (EDM). As a lab we are both a wet and dry lab where we design wet lab experiments that maximize the capabilities of our mathematical methods. The results from this data driven science approach then allows us to generate mechanistic hypotheses that can be again tested experimentally for empirical confirmation. This approach merges traditional hypothesis driven science and the more modern Data driven science approaches into a single virtuous cycle of discovery.

ジェラルド・パオ
准教授
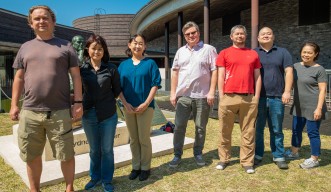
生物システムユニット
生物システムユニットでは、微生物が廃棄物を分解しその過程でエネルギーを放出する装置の研究を行っています。この取り組みは、泡盛蒸留所、養豚および養鶏場、砂糖工場、...

イゴール・ゴリヤニン
教授(アジャンクト)

生物デザインユニット
生物デザインユニットは、生物の発生や形態機能が、気候変動の中でどのように進化していくのかを研究しています。

中山 尚美
教授
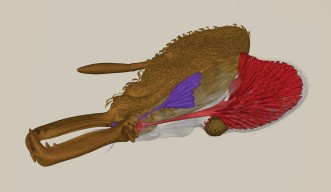
生物多様性・複雑性研究ユニット
生物多様性・複雑性研究ユニットは、生態学的・進化的プロセスがどのように生物多様性を生み出し維持しているのか、また、それらのプロセスが人間活動によってどのように変化しているのかを探っています。

エヴァン・エコノモ
教授(アジャンクト)
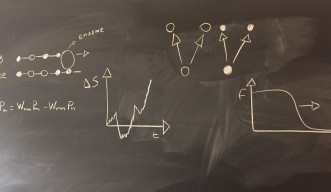
生物複雑性ユニット
生物複雑性ユニットでは、生体分子回路から細胞集団までの様々な生物物理学システムにおいてどのように確率的変動下でも正常に機能しているのかを対象に研究をしています。

シモーネ・ピゴロッティ
教授
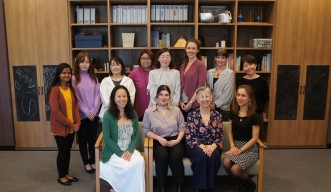
発達神経生物学ユニット
発達神経生物学ユニットでは、注意欠如多動症(ADHD)における動機付けプロセスの特性、日常生活への影響、および社会心理的支援についての研究を行っています。

ゲイル・トリップ
教授

知覚と行動の神経科学ユニット
マウス脳において行動コンテキストが、どのように臭覚情報処理を調整するかを研究しています。手法は、神経生理学、イメージジング、回路解析、行動科学などを用います。

福永 泉美
准教授
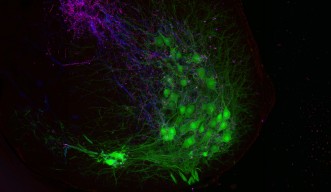
神経回路ユニット
神経回路ユニットでは、分子生物学、マウス遺伝学、電気生理学、光遺伝学、行動解析などを用いて、運動系の神経回路の研究を進めています。

吉田 富
教授
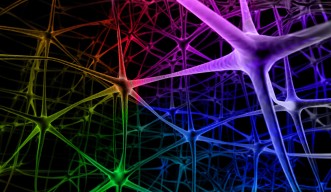
神経情報・脳計算ユニット
感覚知覚、学習と記憶、および意思決定などの脳の認知機能は、神経回路による計算から出現します。神経情報・脳計算ユニットでは、機械計算と比較して生物学的神経計算が有...

深井 朋樹
教授

神経活動リズムと運動遂行ユニット
脳活動の時間的シーケンスは、どのようにして身体の動きに変換されるのだろうか?私達は様々なツールを用いて多角的に、小脳回路の神経リズムの中にその答えを求めている。

マリルカ ヨエ・ウーシサーリ
准教授

神経生物学研究ユニット
神経生物学研究ユニットの目標は、脳における学習の神経メカニズムを解明することです。本ユニットは学習経験の結果シナプスで起こる物理的な変化、またこれらの変化が、動...

ジェフ・ウィッケンス
教授
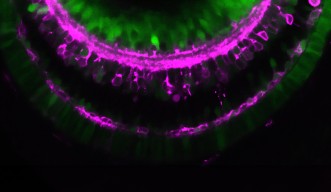
神経発生ユニット
神経発生ユニットでは、ゼブラフィッシュの網膜をモデルに、神経細胞の分化、神経回路形成、神経変性と神経再生を制御するメカニズムについて研究しています。

政井 一郎
教授
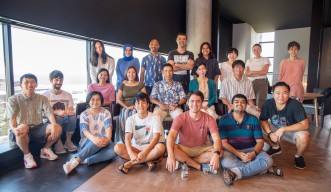
神経計算ユニット
神経計算ユニットでは、脳のように柔軟かつ確実な学習を実現するアルゴリズムの開発と、それによる脳の学習の仕組みの解明に取り組んでいます。最大のテーマは「強化学習」...

銅谷 賢治
教授
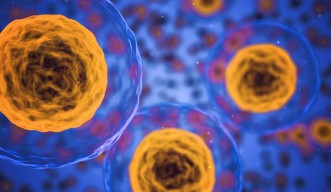
細胞シグナルユニット
細胞シグナルユニットでは、マウスモデルを用いながら、がん、神経疾患、免疫疾患、および糖尿病、肥満などの様々な疾患の原因を分子レベルで解明しようとしています。その...

山本 雅
教授

細胞分裂動態ユニット
細胞分裂動態ユニットでは、ヒト培養細胞とメダカ初期胚を用いて、遺伝情報の継承と初期発生の仕組みについて、特に紡錘体に焦点を当てて研究しています。

清光 智美
准教授

細胞増殖・ゲノム編集ユニット
Every day, millions of cells in our body divide to maintain essential tissue functions. Errors in cell division can lead to developmental disorders or cancer. The research of the unit is focused on molecular mechanisms of cell divison and quality control in normal and cancer cells to understand tumor-suppressive mechanisms and identify biomarkers that confer a cancer-specific vulnerability to chemical drugs. The unit combines high throughput imaging, gene editing and genome wide screens to open new avenues for therapeutic development.

フランツ・マイティンガー
准教授